Social Aspects of Success and Failure in Cultural Markets
This week on ATP, Marco and John had a discussion about Flappy Bird, the irritatingly addictive and unexpectedly successful iOS game that was pulled from the App Store by its developer at the height of its popularity. The hosts’ views shifted around a little during the discussion, but I think it’s fair to say that they had a basic difference of opinion. Marco thought that, at bottom, the game succeeded for good reasons having to do with its own design. As he described it in a related post,
Flappy Bird’s success was hilarious, but it also appears to be completely earned. I’ve read the posts suggesting he cheated at the ranks or reviews, but I haven’t seen any that supported those claims enough. … A charming, comically shitty, addictive, accessible yet difficult, very casual, very quick to play, completely free game with no manipulative in-app purchases? Of course it succeeded in the App Store, fair and square.
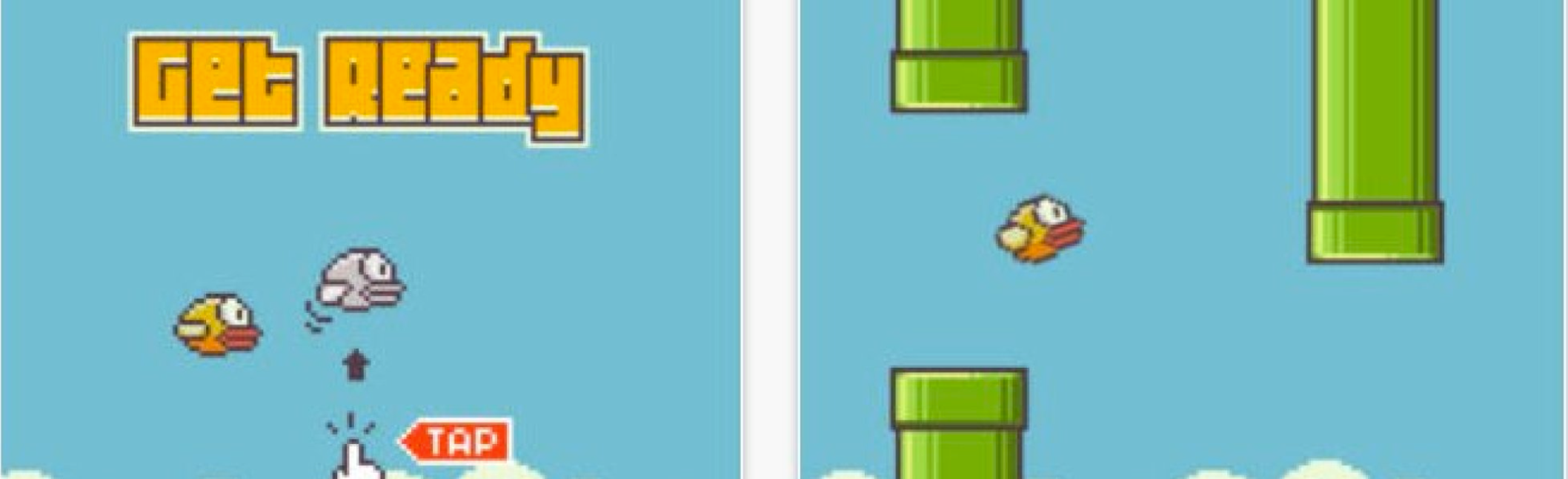
High Score: 1
John, meanwhile, thought that before it exploded onto the scene, there were so many games both similar in kind and at least as good (or better) in quality to Flappy Bird that its success was best understood as a kind of accident, a consequence of the unpredictable dynamics of “meme pools” or cultural markets where popularity can cascade in a way that is rapid, unpredictable, and largely independent of how deserving the successful item is. He likened it to the familiar image of a butterfly flappying—sorry, flapping—its wings and chaotically producing a thunderstorm elsewhere.
As I say, the discussion shifted around, and some middle ground emerged as it went on, so I don’t want to caricature the hosts’ views. But between them John and Marco expressed an old puzzle about the connection between popularity and quality. In many markets—especially markets for cultural goods like music, film, books, and latterly computer games—we see a phenomenon where the most successful goods are way, way more successful than the median product in the market. Now, you might think (and many do think) that this suggests there must be something about those leading products that differentiates them from their competitors and explains their success. It might be “quality” in some abstract sense, or more often maybe a quality or feature that we can point to. Maybe it’s addictive, or compelling, or excellent brain candy, or what have you. And yet, despite the fact that leading goods in these markets are vastly more successful than their competitors, and despite the fact that this keeps happening, it is extremely difficult to reliably predict which book or song or game will be the next runaway best-seller. This is captured in phrases like “all hits are flukes” (from the world of TV shows) or in William Goldman’s remark that, when it comes to the entertainment industry, “Nobody knows anything”. Proven talent fails to deliver, sure-fire hits flop, a low-budget effort becomes a huge hit—although, crucially, many other low-budget efforts are consigned to oblivion, too.

A lot of movies get made
Sociologists are very interested in this phenomenon. As you might imagine, it is hard to study in a rigorous way, especially when what’s at issue is the large-scale success or failure of independently produced cultural objects. But in recent years, it’s become possible to attack this problem in new ways. A few years ago, sociologists Matt Salganik, Duncan Watts and Peter Sheridan Dodds published a very nice paper in Science titled “Experimental study of inequality and unpredictability in an artificial cultural market.” Here’s the abstract:
Hit songs, books, and movies are many times more successful than average, suggesting that “the best” alternatives are qualitatively different from “the rest”; yet experts routinely fail to predict which products will succeed. We investigated this paradox experimentally, by creating an artificial “music market” in which 14,341 participants downloaded previously unknown songs either with or without knowledge of previous participants’ choices. Increasing the strength of social influence increased both inequality and unpredictability of success. Success was also only partly determined by quality: The best songs rarely did poorly, and the worst rarely did well, but any other result was possible.
What they did was set up a music-sharing website that allowed people to download songs. The key experimental manipulation was that some users were presented with a table of links to songs, with no information about how many times the songs had been downloaded. A second group of users saw the same table of links, but ordered by number of previous downloads:
We report the results of two experiments in which we study the outcomes for 48 songs by different bands (18). In both experiments, all songs started with zero downloads (i.e., all initial conditions were identical), but the presentation of the songs differed. In the social influence condition in experiment 1, the songs, along with the number of previous downloads, were presented to the participants arranged in a 16x3 rectangular grid, where the positions of the songs were randomly assigned for each participant (i.e., songs were not ordered by download counts). Participants in the independent condition had the same presentation of songs, but without any information about previous downloads. In experiment 2, participants in the social influence condition were shown the songs, with download counts, presented in one column in descending order of current popularity. Songs in the independent condition were also presented with the single column format, but without download counts and in an order that was randomly assigned for each participant. Thus, in each experiment, we can observe the effect of social influence on each song’s success, and by comparing results across the two experiments, we can measure the effect of increasing the “strength” of the relevant information signal.
The finding is that even this weak information about social influence (the number of download counts) not only increases inequality in the market—i.e. that songs known to be popular become more popular as a result of that knowledge—but increases unpredictability as well. There were big returns to being known to be popular, but which songs became popular was very sensitive to initial conditions. Here is Figure 3 from the paper:

Quality, success, and uncertainty
As the authors remark,
Figure 3 displays the market share (left column) and market rank (right column) of each song in each of the eight social influence worlds as a function of its “quality”" (i.e., its market share and rank, respectively, in the independent condition). Although, on average, quality is positively related to success, songs of any given quality can experience a wide range of outcomes (Fig. 3). In general, the “best” songs never do very badly, and the “worst” songs never do extremely well, but almost any other result is possible. Unpredictability also varies with quality—measured in terms of market share, the “best” songs are the most unpredictable, whereas when measured in terms of rank, intermediate songs are the most unpredictable (this difference derives from the inequality in success noted above). Finally, a comparison of Fig. 3, A and C, suggests that the explanation of inequality as arising from a convex mapping between quality and success (9) is incomplete. At least some of the convexity derives not from similarity of pre-existing preferences among market participants, but from the strength of social influence.
It’s important to note that the phenomenon observed here is not quite the same as a simple cascade of information leading to popularity. In effect, re-running the conditions where social information is available results in the same general patterns but not the same ordering of particular items:
On the one hand, the more information participants have regarding the decisions of others, the greater agreement they will seem to display regarding their musical preferences; thus the characteristics of success will seem predictable in retrospect. On the other hand, looking across different realizations of the same process, we see that as social influence increases (i.e., from experiment 1 to experiment 2), which particular products turn out to be regarded as good or bad becomes increasingly unpredictable, whether unpredictability is measured directly (Fig. 2) or in terms of quality (Fig. 3). We conjecture, therefore, that experts fail to predict success not because they are incompetent judges or misinformed about the preferences of others, but because when individual decisions are subject to social influence, markets do not simply aggregate pre-existing individual preferences. In such a world, there are inherent limits on the predictability of outcomes, irrespective of how much skill or information one has.
In short, Marco is right that Flappy Bird would likely not have succeeded as it did without having the qualities that he listed. Games like Flappy Bird will occasionally be catapulted to success in the App Store, and when they are it will be pretty clear, in one sense, what it is about them that made them so popular. But John is right that there are likely many, many games with these qualities in the App Store, and in a market for cultural goods where phenomenally rapid word-of-mouth success can happen, it is next to impossible to predict which of the many candidate games specifically will make it big. This is one reason, by the way, that players in markets for cultural goods often seek to control the distribution pipeline (in more and less legitimate ways) rather than invest too much time and money in content creation. It’s more predictable. This may also help explain why the immediate reaction of some people was to suggest that the developer had gamed the rankings to drive up popularity.
To reiterate, the two variables of interest are inequality of success—the “winner-take-all” aspect of these markets—and uncertainty about which cultural goods will be the ones to take it all. The strength of the experimental work reported in this paper is that it pulls apart these two variables. Because of the way the experiments are designed, the authors have a grip on how “good” the songs are before they cascade to popularity, which is something that is typically not observable ex ante in real markets. And the finding is that, once social influence is introduced, not only does the inequality-generating “critical mass” or “cascade” happen, but we cannot accurately predict which goods it will happen to. Commentators on cultural markets are generally familiar with the first point, because it is undeniably true. But they are often very reluctant to admit the second point. The closest they tend to get is the idea that there’s a two-stage process where baseline quality wins the “initial” competition, and then things are as it were “handed over” to the cascade-generating process. But this is not what the data suggest.
There are probably a range of reasons for people’s reluctance to agree that true unpredictability is a real feature of these markets. Psychologically, people are often predisposed to believe in some version of a Just World Hypothesis where people fundamentally get what they deserve. A little more sociologically—as noted by Salganik et al—the sheer fact of a winner’s huge success focuses attention on the particular features of the good, and encourages people to tell a story about why those features drove it to the top. This story is of course very plausible ex post precisely because the good succeeded so well. The commentariat in the business press, and sadly a great deal of research on management and leadership in business, is built on some version of this error. Seeking an explanation for success, you look only at the successful cases and thus ignore the possibility that the predictors of success you discover are also present in many of the failed cases. In research design this mistake is called sampling on the dependent variable. As John said repeatedly during the show, this will lead you to confuse necessary and sufficient causes.
If you’re interested in learning more about the line of work described here, check out Matt Salganik’s page on the project, where you can read more articles from the experiments and even download the data to play with, if you like. Duncan Watts also has an accessible discussion of this and related ideas in his book Everything is Obvious.